Redis OM 基本教程¶
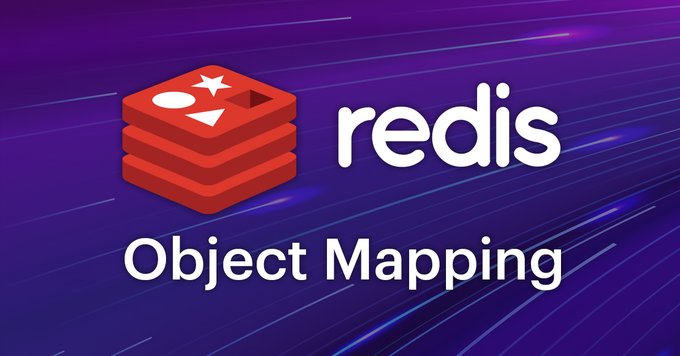
Note
本文翻译自 Redis OM Python 的项目文档 , 有删改。
Redis OM 提供了高层次的抽象, 它可以让当今的 Python 应用更容易地查询 Redis 中储存的数据并为之建模。
本次释出的预览版本提供了以下特性:
为 Redis 对象提供声明式对象映射
声明式二级索引生成
非常丰富多样的 Redis 查询 API
安装 Redis OM¶
你可以通过执行以下命令来安装 Redis OM :
# 通过 PIP 安装
$ pip install redis-om
# 通过 Poetry 安装
$ poetry add redis-om
此外, Redis OM 的某些高级功能需要用到 RediSearch 和 RedisJSON 这两个开源 Redis 模块, 你可以在有需要时酌情安装它们。
除了 Python 版本之外, Redis OM 还提供 .Net 、 Node.js 和 Java(Spring) 版本。
为数据建模¶
Redis OM 包含强大的声明式模型, 以便验证、序列化你的数据并将其持久化至 Redis 。
下文将演示如何通过 Redis OM 建模客户数据。
首先,
我们需要创建一个 Customer
模型:
import datetime
from typing import Optional
from pydantic import EmailStr
from redis_om import HashModel
class Customer(HashModel):
first_name: str
last_name: str
email: EmailStr
join_date: datetime.date
age: int
bio: Optional[str]
之后我们将通过 Customer
模型,
将客户数据储存至 Redis :
import datetime
from typing import Optional
from pydantic import EmailStr
from redis_om import HashModel
class Customer(HashModel):
first_name: str
last_name: str
email: EmailStr
join_date: datetime.date
age: int
bio: Optional[str]
# 首先,创建一个新的 Customer 对象:
andrew = Customer(
first_name="Andrew",
last_name="Brookins",
email="andrew.brookins@example.com",
join_date=datetime.date.today(),
age=38,
bio="Python developer, works at Redis, Inc."
)
# 模型将在无需与 Redis 通讯的情况下,自动创建一个全局唯一的主键:
print(andrew.pk)
# > "01FJM6PH661HCNNRC884H6K30C"
# 之后我们可以通过调用 save() 方法将模型储存到 Redis 里面:
andrew.save()
# 要通过主键获取相应的数据,我们只需要调用 Customer.get() 方法即可:
assert Customer.get(andrew.pk) == andrew
就这么简单!
如果你想要学习更多 Redis OM 的查询执行方法, 那么可以阅读我们的 Getting Started Guide 文档。
或者你也可以接着读下去, 学习如何通过 Redis OM 进行数据验证。
通过模型验证数据¶
Redis OM 使用了 Pydantic , 它可以基于你在模型类中为字段添加的类型注解进行数据验证。
因为 Customer
模型的 first_name
字段被标记成了 str
类型,
所以数据验证会保证该字段的只总是字符串。
此外,
由于每个 Redis OM 模型同样也是 Pydantic 模型,
所以你还可以使用诸如 EmailStr
、 Pattern
等等更为复杂多样的 Pydantic 校验器!
比如说,
由于我们将 email
字段标记成了 EmailStr
类型,
所以程序在尝试使用不正确的邮箱地址创建 Customer
实例时将引发一个验证错误:
import datetime
from typing import Optional
from pydantic import EmailStr, ValidationError
from redis_om import HashModel
class Customer(HashModel):
first_name: str
last_name: str
email: EmailStr
join_date: datetime.date
age: int
bio: Optional[str]
try:
Customer(
first_name="Andrew",
last_name="Brookins",
email="Not an email address!",
join_date=datetime.date.today(),
age=38,
bio="Python developer, works at Redis, Inc."
)
except ValidationError as e:
print(e)
"""
pydantic.error_wrappers.ValidationError: 1 validation error for Customer
email value is not a valid email address (type=value_error.email)
"""
任何现存的 Pydantic 校验器都能够无缝用作 Redis OM 模型的类型注解, 当然你也可以自行编写任意复杂的定制校验器。
要了解更多校验器的用法, 请阅读 Data Validation 文档.
多样查询和嵌入式模型¶
无论你以何种方式运行 Redis , 上述展示的数据建模、验证和保存操作应该都能够正常作用于 Redis 。
接下来, 我们将向你展示 Redis OM 提供的多样查询表达式和嵌入式模型 功能。 这些功能要求你在 Redis 的部署环境中安装了 RediSearch 和 RedisJSON 两个模块, 又或者你运行的是 Redis Enterprise 版本。
查询¶
Redis OM 提供了一套多样查询语言(rich query language), 以便你通过 Pyhton 表达式来查询 Redis 数据。
为了展示多样查询语言是如何运行的,
我们需要对前面定义的 Customer
模型做一些修改。
通过为 last_name
字段和 age
字段添加语句 Field(index=True)
,
以此来告知 Redis OM 我们想要为这两个字段添加索引:
import datetime
from typing import Optional
from pydantic import EmailStr
from redis_om import (
Field,
HashModel,
Migrator
)
class Customer(HashModel):
first_name: str
last_name: str = Field(index=True)
email: EmailStr
join_date: datetime.date
age: int = Field(index=True)
bio: Optional[str]
# 现在,在一个已经安装好了 RedisSearch 模块的 Redis 环境中,
# 我们可以执行以下查询……
# 在执行查询之前,我们需要执行迁移操作以便建立 Redis OM 将要用到的索引。
# 除了这里展示的方法之外,你还可以通过 CLI 工具中的 migrate 命令来完成这一操作。
Migrator().run()
# 查找所有姓氏为 "Brookins" 的客户
Customer.find(Customer.last_name == "Brookins").all()
# 查找所有姓氏不为 "Brookins" 的客户
Customer.find(Customer.last_name != "Brookins").all()
# 查找所有姓氏为 "Brookins" 或者年龄为 100 又或者姓氏为 "Smith" 的用户
Customer.find((Customer.last_name == "Brookins") | (
Customer.age == 100
) & (Customer.last_name == "Smith")).all()
上述展示的这些查询——以及 Redis OM 支持的其他多样查询——它们之所以能够成功执行, 完全是由于 Redis OM 为我们自动完成了管理索引的工作。
索引查询所使用的多样表达式语法参考了 Django ORM 、 SQLAlchemy 和 Peewee 的语法, 我相信你会喜欢它们的!
嵌入式模型¶
Redis OM 可以让你像使用文档数据库那样对嵌套模型执行查询和储存操作, 并且不失 Redis 的强大和快速。 让我们看看它是如何做到这一点的。
在下面这个例子中,
新定义的 Address
模型将嵌入到 Customer
模型里面:
import datetime
from typing import Optional
from redis_om import (
EmbeddedJsonModel,
JsonModel,
Field,
Migrator,
)
class Address(EmbeddedJsonModel):
address_line_1: str
address_line_2: Optional[str]
city: str = Field(index=True)
state: str = Field(index=True)
country: str
postal_code: str = Field(index=True)
class Customer(JsonModel):
first_name: str = Field(index=True)
last_name: str = Field(index=True)
email: str = Field(index=True)
join_date: datetime.date
age: int = Field(index=True)
bio: Optional[str] = Field(index=True, full_text_search=True,
default="")
# 创建嵌套模型
address: Address
# 在拥有以上两个模型并且在 Redis 环境中安装了 RedisJSON 模块的情况下,
# 我们可以执行以下查询……
# 执行迁移操作以便建立 Redis OM 将要用到的索引
Migrator().run()
# 查找所有居住在 San Antonio, TX 的客户
Customer.find(Customer.address.city == "San Antonio",
Customer.address.state == "TX")
更多¶
关于 Redis OM 更详细的用法, 请查阅项目文档。